Of all the NBA season awards, voting the most improved player seems to be the worst defined one. The reason therefore is that improvement can happen on so many levels (for those of you that are interested in a good read about the different ways to look at it, check out
this article by Rob Mahoney). It is also complicated to keep your eye on the complete picture, as you have to look at two or more years at the same time.
To give at least a data-based picture of potential candidates, I will use the data from
Basketball-Reference. The focus will be on minutes independent data, as in my opinion improvement has to be more than just an increase in minutes played. Today, I will present the candidates and in part II (hopefully tomorrow) I will take a closer look at what they improved. Feel free to let me know where you agree or disagree (my dear hypothetical reader)
Filtering for candidates
To be a potential iMIP (imho MIP) candidate, players have to fulfill the following minutes/games criteria:
- Play at least 8 minutes per game in at least 41 games in 2012-13 (henceforth called 2013)
- Play at least 18 minutes per game in at least 41 games in 2013-14 (henceforth called 2014)
This means (more or less) that the player was last year at least a borderline rotation guy and is this season at least a 7th man (second player coming from the bench) and not only playing in garbage time.
Furthermore, I use
Player efficiency rating (PER) to summarize the qualities of a player. There are certainly disadvantages to PER, but its advantage is that it is pace and playing time adjusted. The thresholds for a player to be available are:
- increased his PER in 2014 at least by 3
- has a PER in 2014 that is at least 15
I am aware that 3 is an arbitrary threshold, but I picked it on the impression that enough players surpassed it. A PER above 15 on the other hand means simply that your performance in 2014 is 'measured' as above average. It's a feel-good story if you improved from being a bench warmer to rotation guy, but it's not necessarily iMIP material. This gives us the following picture:
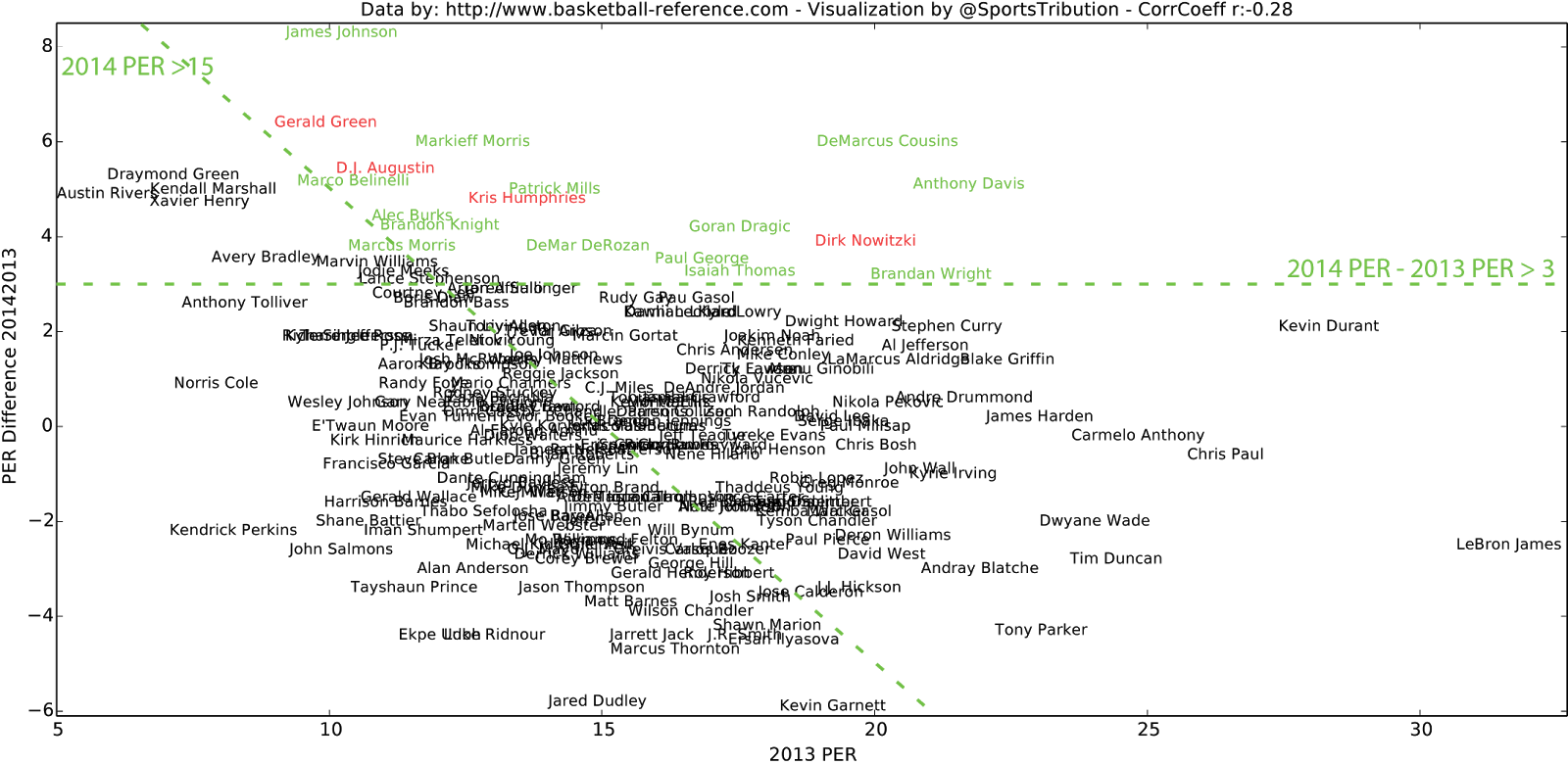 |
click image to enlarge |